Expo
view channel
view channel
view channel
view channel
view channel
view channel
Medical Imaging
AICritical CareSurgical TechniquesPatient CareHealth ITPoint of CareBusiness
Events
- Point-Of-Care EEG System Significantly Reduces Length of Patient Stay in ICU
- High-Quality Thin Film Conductor Paves Way for Wearable Electronics with Longer Life Batteries
- Intraoral Camera for Cancer Screening Eliminates Guesswork in Interpreting Clinical Findings
- AI Algorithm Non-Invasively Measures Intracranial Pressure in ICU Patients Following Traumatic Brain Injury
- Healthcare Device Powered By Body Heat Marks First Step Toward Battery-Free Wearable Electronics
- Novel Fluorescent Imaging Agent Helps Surgeons Visualize Missed Lung Tumors
- EHR–Based Nudge Intervention for Surgeons to Reduce Breast Cancer Overtreatment
- Novel Electrosurgical Device Could Be a Game-Changer for Breast Cancer Treatment
- New Robotic Navigation Platform Provides Surgeons Best-In-Class Solution for Orthopedic Treatment
- Breakthrough AR Surgical System Brings Precision-Enhanced Visualization into the OR
- Surgical Capacity Optimization Solution Helps Hospitals Boost OR Utilization
- Game-Changing Innovation in Surgical Instrument Sterilization Significantly Improves OR Throughput
- Next Gen ICU Bed to Help Address Complex Critical Care Needs
- Groundbreaking AI-Powered UV-C Disinfection Technology Redefines Infection Control Landscape
- Clean Hospitals Can Reduce Antibiotic Resistance, Save Lives
- International Hospital Federation Awards 2024 Finalists Announced
- 2024 World Medical Tourism Conference and Medical Tourism Expo to Showcase Latest Innovations
- BD Acquires Edwards Lifesciences' Critical Care Product Group for USD 4.2 Billion
- MEDICA INNOVATION FORUM for the Healthcare Innovations of the Future
- Johnson & Johnson Acquires Cardiovascular Medical Device Company Shockwave Medical
- Strategic Collaboration to Develop and Integrate Generative AI into Healthcare
- AI-Enabled Operating Rooms Solution Helps Hospitals Maximize Utilization and Unlock Capacity
- AI Predicts Pancreatic Cancer Three Years before Diagnosis from Patients’ Medical Records
- First Fully Autonomous Generative AI Personalized Medical Authorizations System Reduces Care Delay
- Electronic Health Records May Be Key to Improving Patient Care, Study Finds
- Cartridge-Based Hemostasis Analyzer System Enables Faster Coagulation Testing
- Critical Bleeding Management System to Help Hospitals Further Standardize Viscoelastic Testing
- Point of Care HIV Test Enables Early Infection Diagnosis for Infants
- Whole Blood Rapid Test Aids Assessment of Concussion at Patient's Bedside
- New Generation Glucose Hospital Meter System Ensures Accurate, Interference-Free and Safe Use
Expo
view channel
view channel
view channel
view channel
view channel
view channel
Medical Imaging
AICritical CareSurgical TechniquesPatient CareHealth ITPoint of CareBusiness
Events
Advertise with Us
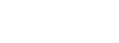
- Point-Of-Care EEG System Significantly Reduces Length of Patient Stay in ICU
- High-Quality Thin Film Conductor Paves Way for Wearable Electronics with Longer Life Batteries
- Intraoral Camera for Cancer Screening Eliminates Guesswork in Interpreting Clinical Findings
- AI Algorithm Non-Invasively Measures Intracranial Pressure in ICU Patients Following Traumatic Brain Injury
- Healthcare Device Powered By Body Heat Marks First Step Toward Battery-Free Wearable Electronics
- Novel Fluorescent Imaging Agent Helps Surgeons Visualize Missed Lung Tumors
- EHR–Based Nudge Intervention for Surgeons to Reduce Breast Cancer Overtreatment
- Novel Electrosurgical Device Could Be a Game-Changer for Breast Cancer Treatment
- New Robotic Navigation Platform Provides Surgeons Best-In-Class Solution for Orthopedic Treatment
- Breakthrough AR Surgical System Brings Precision-Enhanced Visualization into the OR
- Surgical Capacity Optimization Solution Helps Hospitals Boost OR Utilization
- Game-Changing Innovation in Surgical Instrument Sterilization Significantly Improves OR Throughput
- Next Gen ICU Bed to Help Address Complex Critical Care Needs
- Groundbreaking AI-Powered UV-C Disinfection Technology Redefines Infection Control Landscape
- Clean Hospitals Can Reduce Antibiotic Resistance, Save Lives
- International Hospital Federation Awards 2024 Finalists Announced
- 2024 World Medical Tourism Conference and Medical Tourism Expo to Showcase Latest Innovations
- BD Acquires Edwards Lifesciences' Critical Care Product Group for USD 4.2 Billion
- MEDICA INNOVATION FORUM for the Healthcare Innovations of the Future
- Johnson & Johnson Acquires Cardiovascular Medical Device Company Shockwave Medical
- Strategic Collaboration to Develop and Integrate Generative AI into Healthcare
- AI-Enabled Operating Rooms Solution Helps Hospitals Maximize Utilization and Unlock Capacity
- AI Predicts Pancreatic Cancer Three Years before Diagnosis from Patients’ Medical Records
- First Fully Autonomous Generative AI Personalized Medical Authorizations System Reduces Care Delay
- Electronic Health Records May Be Key to Improving Patient Care, Study Finds
- Cartridge-Based Hemostasis Analyzer System Enables Faster Coagulation Testing
- Critical Bleeding Management System to Help Hospitals Further Standardize Viscoelastic Testing
- Point of Care HIV Test Enables Early Infection Diagnosis for Infants
- Whole Blood Rapid Test Aids Assessment of Concussion at Patient's Bedside
- New Generation Glucose Hospital Meter System Ensures Accurate, Interference-Free and Safe Use